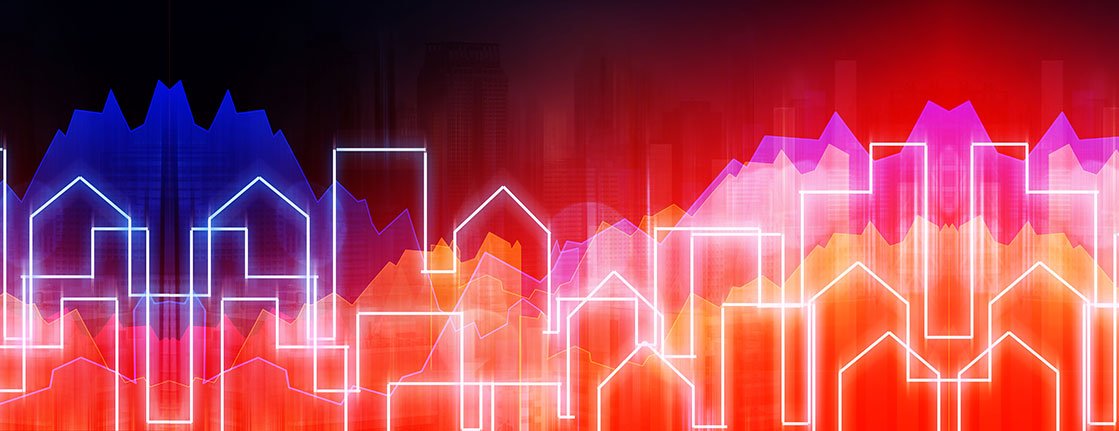
Declining credit demand and an increasing focus from regulators means lenders need to look internally for more effective ways to grow their business without increasing their level of risk. With a greater influx of data and rapid changes in consumer behaviour, there is an increasing need for lenders to look beyond traditional models to predict default risk. They are now focusing on how they can use the latest machine learning techniques to generate better scores and increase the profitability for their organisation.
While the results of traditional models (such as linear regression) are easy to explain to customers, they are not the most accurate for predicting credit risk for complex applications involving multiple data sources and relationships. On the other hand, newer models of machine learning (such as neural networks) tend to be significantly better at predicting credit risk, but the results are more difficult to interpret and explain.
Equifax has recently obtained the US patent for a new machine learning model called NeuroDecision® Technology, which combines the speed and accuracy of a neural network with the transparency and explainability of traditional linear models. The result is a unique credit risk model that helps lenders understand how credit scoring decisions are determined, so they can stay compliant and make informed decisions when assessing credit risk.
Unlike many neural networks, the Equifax model has been designed to be seamlessly deployed in the lender’s existing environment, providing opportunities for greater automation and faster decision making. This can not only reduce costs, but provide a more predictive credit scorecard that allows lenders to maintain business growth and reduce credit risk.
Recently, a leading bank in the Asia Pacific region approached Equifax to see if our machine learning model was better at predicting credit risk than their own internal scorecard, while also being easy to explain. The bank used the Proof of Concept to determine its level of investment in machine learning technology.
Equifax curated a global team of analytics, modelling and project experts to assess the client’s mortgage application scorecard and analyse 12 months of data and behavioural variables. We also applied our bureau data to understand the performance of declined applications, helping the lender improve the accuracy of predicting the likelihood of customers defaulting on payments, or becoming a credit-worthy customer in the future.
Combining our bureau data with the neural network model provided the bank with the ability to monitor and improve scorecard performance over time. Specifically, results showed that implementing the Proof of Concept would allow the bank to:
- either reduce declines by 15% or bad debt by 4.1%, for the same debt profile
- increase accepted credit applications by 6.6%
- identified 22% more high-risk applicants in the lowest 5% of scores than the benchmark
- enjoy significant gains in sub-segments, including high LVR (26%) and rural (13%) customers.
In the wake of the banking Royal Commission, lenders are looking for a credit assessment model that is both accurate, transparent and easy to explain, particularly as data is collected from an increasing number of sources. The use of new machine learning models like NeuroDecision® Technology is likely to become a linchpin to lenders over the next decade, providing a key differentiator between those who have the technology, and those that do not.
Disclaimer: The information, materials and opinions contained on this article are for general information purposes only, are not intended to constitute legal, financial or other professional advice and should not be relied on or treated as a substitute for specific advice relevant to particular circumstances. We make no warranties, representations or undertakings about NeuroDecision® Technology (including, without limitation, any as to the quality, accuracy, completeness or fitness for any particular purpose of that product in the context of your requirements or needs). The performance of NeuroDecision® Technology in the referenced case study is not necessarily a reliable indicator of future results in a different context.
Related Posts
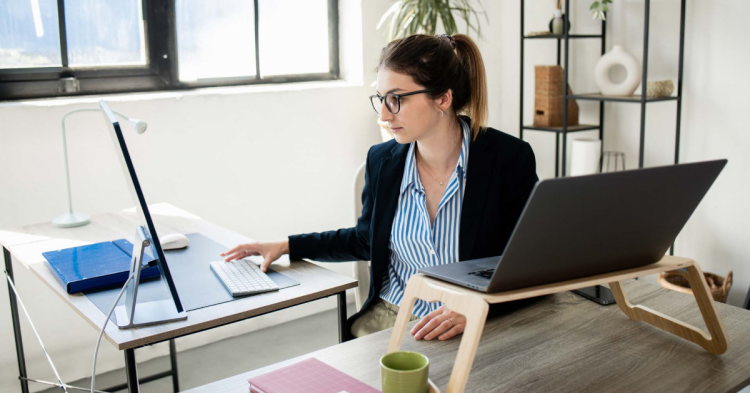
The Anti-Money Laundering and Counter-Terrorism Financing (AML/CTF) reforms are crucial for protecting the financial system from illegal activities. This overview explains the key changes brought about by Tranche 2 detailing essential compliance mandates and effective risk management practices.