How Do You Know If You're Using Quality Data?
Wherever there is data, there are answers. But there are right answers, and there are wrong answers.
Good-quality data can be relied upon to give valuable insights for better decision making, but the same can't be said of low-quality data. Data that is incomplete, dated or not fit for purpose can result in inaccurate or misleading outcomes.
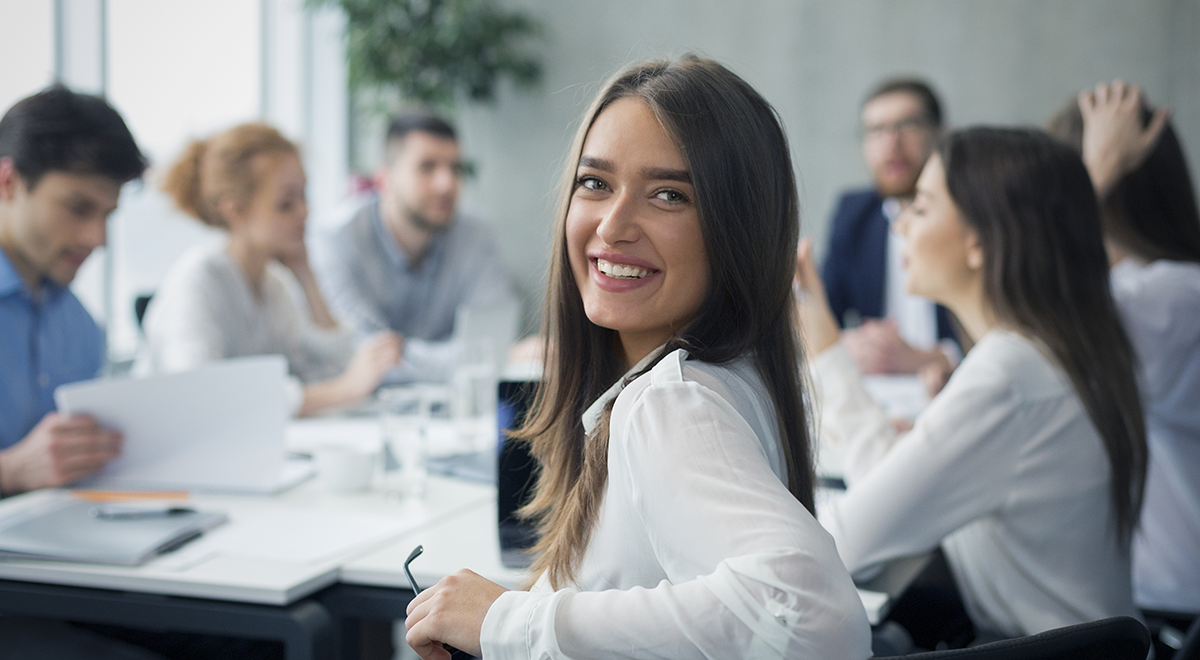
A business decision based on flawed information can alienate customers and cost organisations dearly in lost revenue, missed opportunities and compensatory overspending. Estimates by Gartner1 place the average cost to organisations of poor data quality at $12.9 million each year. Bad data equals wrong answers.
Data quality is a gamechanger for building competitive advantage in today's digital, customer-driven economy. With more data available than ever, it is a business imperative to find value in this sea of information. In the financial services industry, the harnessing of newly available income and expenditure data, for example, offers the opportunity to improve credit risk and segment customer bases to deliver tailored products for greater brand loyalty and financial literacy.
When the Economist2 announced in 2017 that the world's most valuable resource is no longer oil but data, organisations were already leveraging data to understand their customers, predict future trends, and unlock new revenue systems to drive business growth. Five years later, there can be little doubt that organisations who don't prioritise the accuracy, completeness, consistency, credibility and currentness of data will be left behind in the global race to be data-driven.
So how can businesses increase their data maturity? A useful starting point is to find out more about the reliability of the data that informs your insights. When seeking to partner with a third-party provider, understand that data quality is not the same from one provider to the next. Be sure to ask these three qualifying questions at minimum to help compare data integrity between different providers.
1. What is the depth and detail of data sources?
Variety matters when it comes to data quality. The more depth of data and breadth of data sources, the better. When sources are limited, and datasets lack relevant detail, there's a good chance that part of the data story is missing. This lack of clarity may lead to inaccurate conclusions.
Diversity and breadth of data brings about a clearer picture, generating insights with greater predictability. Consider the example of a commercial credit report. For it to give you the information you need to reduce risk exposure, it should draw on enough data points to detail who is making the enquiry, the date, the dollar value, the type of credit and the industry it originated from. When any of these fields are missing, it's a clue that the report is probably based predominantly on ASIC search data and lacks the associated context to interpret warning signs of credit risk.
For a credit report to properly tell a story based on data, ensure your provider draws on a wide breadth of reliable, authoritative sources that span consumer, commercial, fraud and identity, and new data sources like bank transaction and Buy Now Pay Later (BNPL).
2. How fresh is the data?
The shelf life of data shrinks as the volume and velocity of data extraction increases. Because data is rendered obsolete or inaccurate the moment newer information becomes available, the more frequently it is refreshed, the more accurate the insights.
Consider the commercial credit report example again, where an increased number of credit enquiries has shown to be a critical early warning of financial distress. Unless the ASIC data your provider uses is timely and up to date, it may miss all-important recent credit enquiries, thus overlooking vital information you can use to inform your risk assessment.
Ask the data provider how regularly they refresh their data. Do they collect and update information in real-time? As the volume of data and the pace of change continues to skyrocket, data management is becoming an increasing challenge for organisations and data providers alike. Yet, in our "always-on" world, it is more important than ever to work with current and quickly accessible data.
Leading the way in building and scaling data management in the financial services industry, Equifax is implementing a data fabric built on the Google Cloud platform. As part of a multi-year digital initiative to transform customer-facing systems and back-end technology, the Equifax data fabric enables consistent, controlled and secure access to a rich palette of data from Cloud or on-premises. This unified environment provides built-in data quality and data governance, placing clean and complete data at the fingertips of business users.
3. How well is data matched?
Two data records might appear dissimilar at first glance, but they turn out to be the same on closer investigation. A data provider's ability to match these source records depends on factors like the quality of their data preparation, the depth and breadth of their data sets and the sophistication of their data-matching technology. Machine learning algorithms must be advanced enough to work across disparate data sets to compare, identify or merge related entities.
By joining the dots on a myriad of information, a more complete view emerges of financial health and customer behaviour. Consider the commercial credit risk example again. When a deep level of insight is required into a business's validity, financial stability and performance, it is crucial to see both the commercial and consumer profiles of the company's directors. Both information sets work together to form a complete picture of potential risk. Correlating and linking business data with information about the people behind the business can reveal critical details about financial instability that may otherwise have gone unnoticed.
The takeaway point is that good quality data drives more informed decisions to increase revenue, reduce costs, solve problems and stimulate growth. Poor quality data has the opposite effect, wasting your resources with bad outcomes and costly errors. Establish a value-driven foundation that delivers the right answers by prioritising data quality in your dealings with data providers.
Download our infographic for 10 key questions to ask when choosing your data partner.
Don't wait until data quality becomes a problem. Get in touch now to learn more about the reliability and predictiveness of Equifax data.
1 https://www.gartner.com/smarterwithgartner/how-to-improve-your-data-quality
2 https://www.economist.com/leaders/2017/05/06/the-worlds-most-valuable-re...
Related Posts
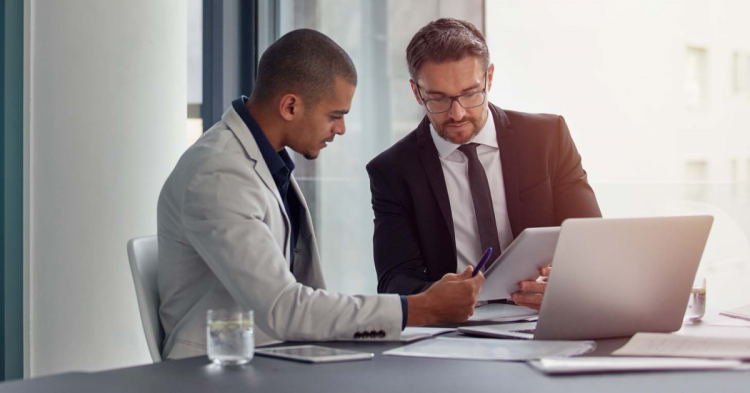
Cleansing your customer data of deceased records improves data integrity and helps businesses mitigate legal and financial risks. As the new year approaches, it’s an ideal time to cleanse your database and ensure it contains accurate and up-to-date customer information.
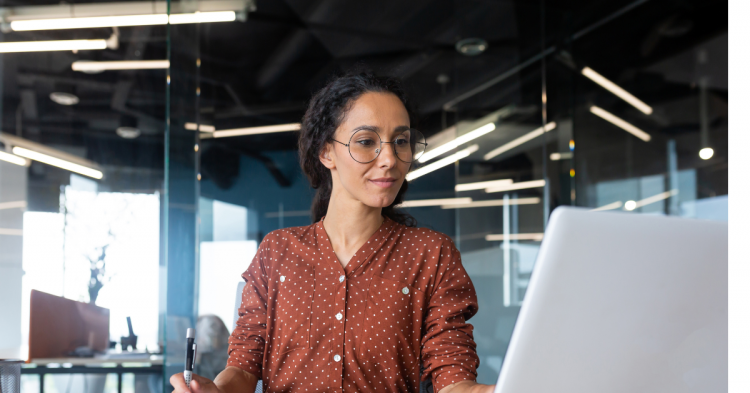
While PEP, sanctions and adverse media screening are vital for customer due diligence, false positives create unnecessary delays and frustration. These inaccurate matches waste time and resources, slowing down onboarding and impacting the customer experience.
So, how can you optimise your screening process and minimise false positives?