Credit Scoring with Alternative Data: The Way Forward
Alternative data opens up a world of possibilities for more accurately determining creditworthiness. Where a person lives, where they dine out and how they spend their income…these are all data sources that have the potential to enhance traditional credit score models for improved assessment of financial reliability.
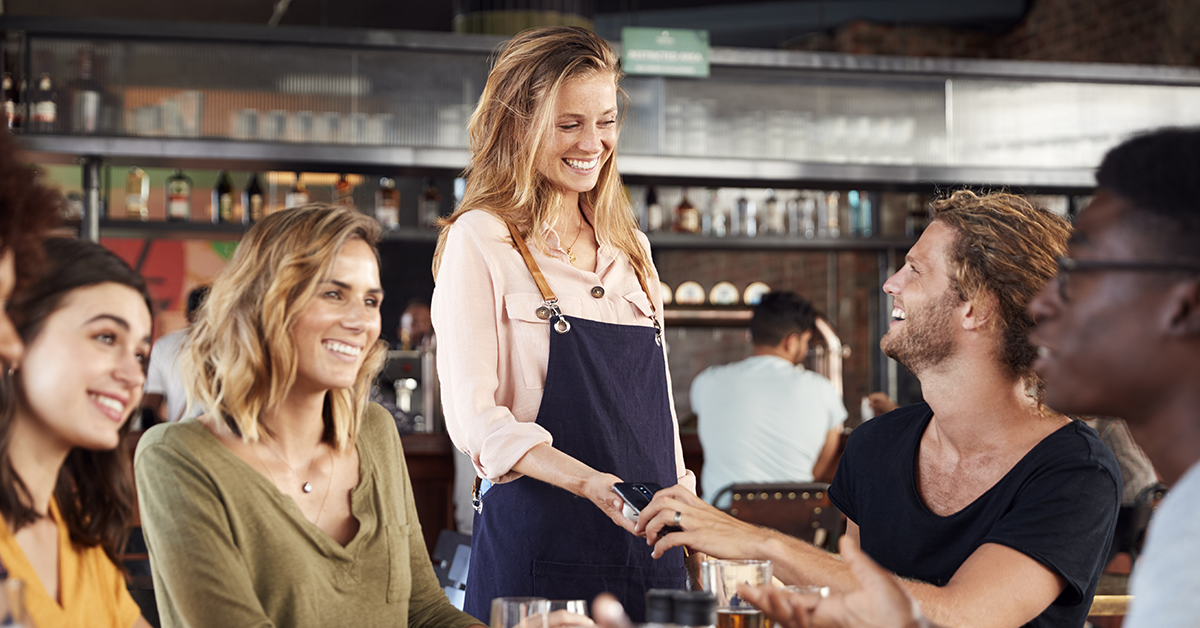
Incorporating alternative data like cash flow analysis, credit card usage, employment background and rental history into the lending process brings benefits for both lenders and consumers. For consumers unable to prove their creditworthiness, these unconventional sources help to extend the pool of data from which lenders can base their decisions. First-time borrowers or immigrants with a limited or non-existent credit history can demonstrate financial responsibility through aggregated information about their address and income stability, saving habits and utility bill payments.
For lenders dealing with marginal, new-to-bureau and “thin” files, increasing the amount of data about an applicant outside of their financial history can fill in the gaps and help to reduce the unknown. With this ability to make more predictive decisions, lenders can expand credit access to previously underserved populations and have the opportunity to increase portfolio profitability.
Alternative data already in use
Already alternative data is making its way into traditional credit scoring models. Geodemographic and Buy Now Pay Later (BNPL) data are currently available as supplements to Equifax’s new generation credit score. Working with non-traditional data that is aggregated and depersonalised, data scientists are using machine learning technology to extract signals of credit risk.
Marcus Bruhn, General Manager of Equifax Data Science, explains: “We analyse the correlations between sets of information such as between the volume of credit enquiries in a region compared to the volume of defaults. With these observations, we create a range of categories that are useful in providing intelligence on what this location’s population is like. For example, the top category would show the greatest ratio of enquiries to defaults, which would indicate to a lender that this region has a lower likelihood of delinquency.”
Richer data sets in the pipeline
The rollout of open banking brings with it many new data sets that have the potential to add another layer of information to existing credit reporting. Work currently underway with bank transaction data hints at the possibilities that lie ahead for non-traditional data sets to increase financial inclusion.
With the help of advanced analytics and innovative techniques, Equifax is currently building a predictive model prototype based on transaction data. Bruhn explains that every transaction tells a story and can reveal patterns and signals indicating credit repayment ability. For example, a high number of account interest payments shows good saving habits, which is a low credit risk indicator. A high number of dishonour or missed payment fees have the opposite effect, indicating a higher risk.
Analysis of income and expense data can add to this picture of financial capability by finding previously unrecognised positive and negative correlations, says Bruhn.
“Discretionary spend is currently grouped by most categorised engines into broad household expenditure categories like ‘restaurants’. But we are drilling down deeper than what these categories provide for. Where you bought your food from – is it from a ‘dine-in’ restaurant or a ‘fast food’ restaurant – these are all indicators that can help supplement traditional credit metrics.”
Ethical credit scoring
Importantly, ethical considerations factor high in how Equifax uses alternative data. A crucial safeguard employed during the development of credit scoring algorithms is the omission of data that could form the basis for unfair bias and discrimination. Also, the rigorous monitoring and testing of models to ensure the detection of any statistical quirks that may subtly bias the results.
Key to the responsible use of data for consumer protection and fair lending is the ability to explain the basis for a credit decision. This explainability is only possible if lenders and consumers understand which variables are driving credit scores. The recent development of NeuroDecisionTM Technology and its use in Equifax’s new generation credit score make it possible for this disclosure to better occur. Unlike traditional linear regression models, this transparency of decision-making does not come at the expense of speed, predictability or coverage. In one consumer case study1, NeuroDecisionTM Technology increased coverage by 7% and improved performance by up to 16%.
With this ground-breaking technology, fairness and ethics can take centre stage as alternative data helps to shape the future of financial inclusion.
Speak to your Account Manager or email us to find out more.
1https://assets.equifax.com/assets/usis/igniteLive2020_ezine.pdf
Related Posts
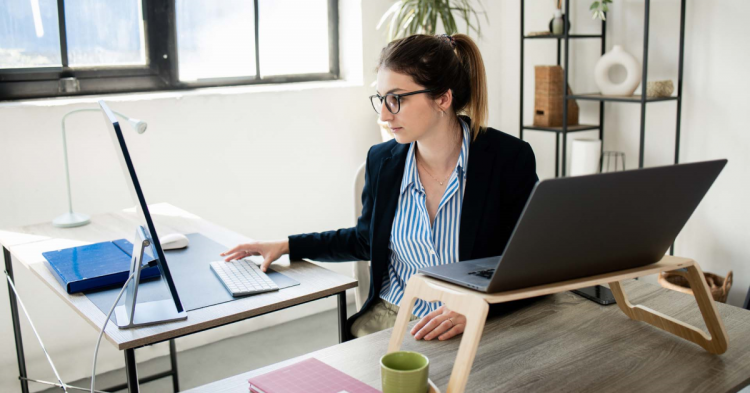
The Anti-Money Laundering and Counter-Terrorism Financing (AML/CTF) reforms are crucial for protecting the financial system from illegal activities. This overview explains the key changes brought about by Tranche 2 detailing essential compliance mandates and effective risk management practices.
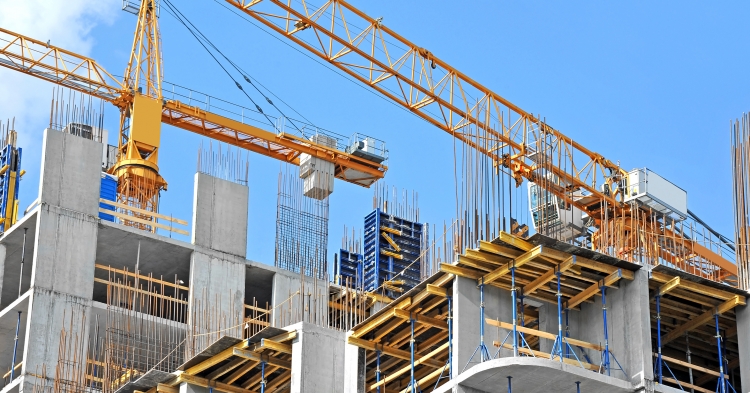
The pace of businesses engaged in construction activity employing more than 5 full time staff entering initial external administration has slowed in the financial year ended June 2024. This is in contrast to the businesses employing less than 5 full time staff which continue to report a higher number of initial external administrations, thus lifting the overall construction business failure levels to record highs.