Can Credit Managers Trust the Quality of their Data?
When it was announced in 2017 that the world’s most valuable resource is no longer oil but data, organisations were already leveraging data to manage credit risk, predict future trends, and unlock new revenue systems to drive business growth.
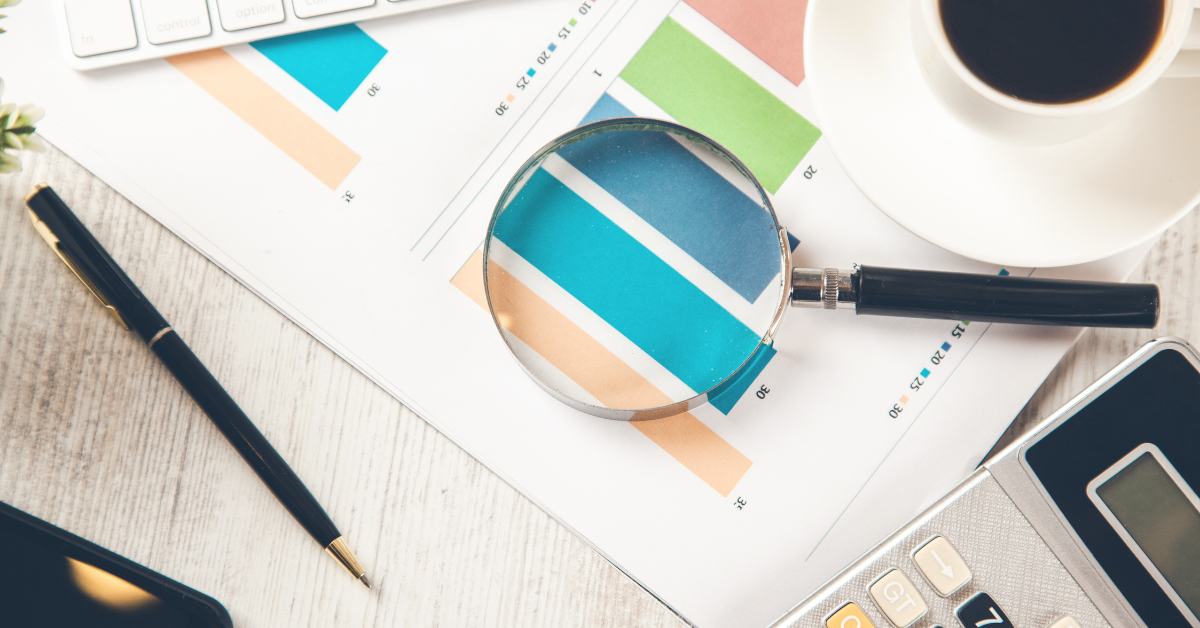
Seven years on, data-based reason and analysis have become part of our everyday. Yet the question must be asked: are we putting too much faith in the infallibility of data? What if the data that underlies our critical and strategic decisions is inaccurate, duplicated or poorly compiled? What if it isn’t fit for purpose or is missing key elements?
Low-quality data undermines decisions
There is a solid argument to be made that today’s business leaders and credit professionals should regard their data integrity with a healthy dose of scepticism. Good-quality data can be relied upon to give valuable insights for better decision-making, but the same can’t be said of low-quality data.
Organisations that don’t prioritise data accuracy, completeness, consistency, credibility and currentness cannot lean into their data as a powerful decision support tool. After all, data that is incomplete, dated or not fit for purpose can cause inaccurate or misleading outcomes and costly long-term errors. Data quality-related problems are likely to lead to missed business opportunities and decisions that fall short of the mark.
A credit decision based on flawed information can alienate customers and cost organisations dearly in lost revenue and compensatory overspending. Estimates by Gartner place the average cost to organisations of poor data quality at $12.9 million each year. Bad data equals wrong answers.
Give attention to data quality
Data quality is a gamechanger for managing risk in today’s highly complex and changeable environment. With more data available than ever, it is a business imperative to find value in this sea of information.
For example, harnessing income and expenditure data opens up a world of possibilities for more accurately determining creditworthiness. For credit professionals dealing with marginal, new-to-bureau and thin files, increasing the amount of data about an applicant outside their financial history can fill in the gaps and reduce the unknown. With this ability to make more predictive decisions, credit professionals can expand credit access to previously underserved populations and increase portfolio profitability.
Integrating high-quality, mission-critical data into these day-to-day credit decisions can give credit professionals the ability to speed the time to ‘yes’, respond quickly to threats and take advantage of opportunities.
Increasing data maturity
So how can businesses increase their data maturity? A useful starting point is to find out more about the reliability of the data that informs your insights. When seeking to partner with a third-party provider, understand that data quality differs from one provider to the next. Be sure to ask these three qualifying questions at a minimum to help compare data integrity between different providers.
1. What is the depth and detail of data sources?
Variety matters when it comes to data quality. The more depth of data and breadth of data sources, the better. When sources are limited and datasets lack relevant detail, there’s a good chance that part of the data story is missing. This lack of clarity may lead to inaccurate conclusions.
Diversity and breadth of data bring about a clearer picture, generating insights with greater predictability. Consider the example of a commercial credit report. For it to give you the information you need to reduce risk exposure, it should draw on enough data points to detail who is making the enquiry, the date, the dollar value, the type of credit and the industry it originated from. When any of these fields are missing, it’s a clue that the report is probably based predominantly on ASIC search data and lacks the associated context to interpret warning signs of credit risk.
For a credit report to properly tell a story based on data, ensure your provider draws on a wide breadth of reliable, authoritative sources that span consumer, commercial, fraud and identity, including bank transactions and Buy Now Pay Later (BNPL).
2. How fresh is the data?
The shelf life of data shrinks as the volume and velocity of data extraction increases. Because data is rendered obsolete or inaccurate the moment newer information becomes available, the more frequently refreshed, the more accurate the insights are.
Consider the commercial credit report example again, where an increased number of credit enquiries has shown to be a critical early warning of financial distress. Unless the ASIC data your provider uses is timely and up to date, it may miss all-important recent credit enquiries, thus overlooking vital information you can use to inform your risk assessment.
Ask the data provider how regularly they refresh their data. Do they collect and update information in real-time? As the volume of data and the pace of change continues to skyrocket, data management is becoming an increasing challenge for organisations and data providers alike. Yet, in our “always-on” world, working with current and quickly accessible data is more critical than ever.
3. How well is data matched?
Two data records might appear dissimilar at first glance, but they turn out to be the same on closer investigation. A data provider’s ability to match these source records depends on factors like the quality of their data preparation, the depth and breadth of their data sets and the sophistication of their data-matching technology. Machine learning algorithms must be advanced enough to work across disparate data sets to compare, identify or merge related entities.
By joining the dots on a myriad of information, a more complete view of financial health and customer behaviour emerges. Consider the commercial credit risk example again. When a deep level of insight is required into a business’s validity, financial stability and performance, it is crucial to see both the commercial and consumer profiles of the company’s directors. Both information sets work together to form a complete picture of potential risk. Correlating and linking business data with information about the people behind the business can reveal critical details about financial instability that may otherwise have gone unnoticed.
The takeaway point is that good quality data drives more informed decisions to increase revenue, reduce costs, solve problems and stimulate growth. Poor quality data has the opposite effect, wasting your resources with bad outcomes and costly errors. Establish a value-driven foundation that delivers the right answers by prioritising data quality in your dealings with data providers.
Contact us today to learn more about the reliability and predictiveness of Equifax data.
Related Posts
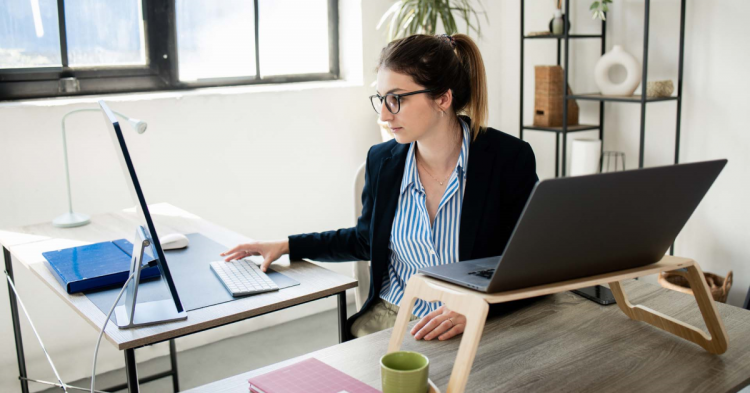
The Anti-Money Laundering and Counter-Terrorism Financing (AML/CTF) reforms are crucial for protecting the financial system from illegal activities. This overview explains the key changes brought about by Tranche 2 detailing essential compliance mandates and effective risk management practices.
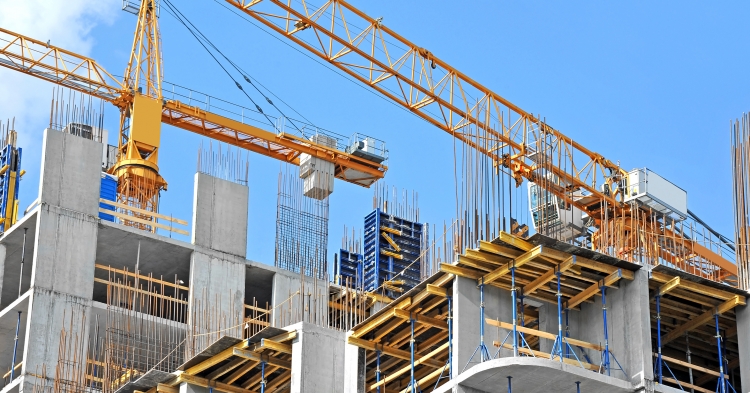
The pace of businesses engaged in construction activity employing more than 5 full time staff entering initial external administration has slowed in the financial year ended June 2024. This is in contrast to the businesses employing less than 5 full time staff which continue to report a higher number of initial external administrations, thus lifting the overall construction business failure levels to record highs.