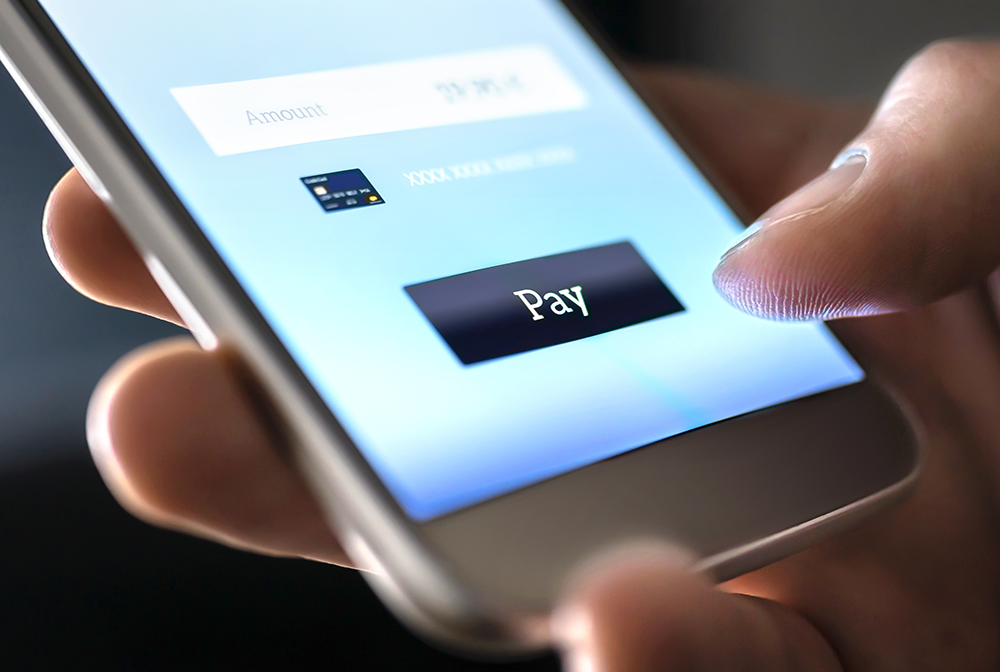
Using our new score, a lender could lower the number of defaults2 in their credit card portfolio by 16.35% and in personal loans by 19.05% compared to our previous generation score. The score can help deliver performance gains in every portfolio: 14.75% for auto loans, 11.54% for residential mortgages and 6.09% for Buy Now Pay Later (BNPL). This increased predictive power maximises risk-adjusted return, allowing lenders to reduce default rates or increase approval rates without taking on more default risk.
So what's behind this stronger, better performing model? Here we explain the innovations and contributing factors that help deliver greater insight into how lenders can improve their underwriting decisions.
1. The point-in-time impact of credit enquiry data has been removed
The new generation scorecard model has been built without the inclusion of 'current' enquiry data. The primary purpose of this change was to ensure the score is more effective and relevant for lenders. While enquiry data is still embedded in the credit report and featured in the credit score, it no longer has a point-in-time impact on the score.
Marcus Bruhn, General Manager Data Science, explains that removing current enquiry data forces the model to use all available information in the credit report rather than making allowances according to where the consumer is submitting their credit application.
"Previously, as soon as a consumer applied for credit at a subprime lender or prime lender, the model would start using this information in its assessment of risk. The model would immediately deduce that an individual who applied for a loan at a subprime lender was riskier than an individual who applied at a prime lender," Bruhn explains.
"Now that current enquiry data is no longer a key dependency for the score to be generated, our new model can base its risk assessment on a broad range of other data. This produces a more effective rank ordering of risk for individual lenders and their portfolios."
Removing current enquiry data also allows for one common score between lenders and consumers. Previously, when an individual applied for credit, the score was immediately affected by the enquiry data. The lender would see this change in the score, but the consumer wouldn't. Now when a consumer checks their online score with a lender that has adopted Equifax’s new generation score, it will be the same score the lender is looking at.
This consistency of a common score is an important step closer towards improved financial literacy. When an individual can see the direct relationship between their score, their actions and better terms of credit, there is a greater understanding of the value in using debt wisely and paying bills on time. Lenders benefit from these more informed customers who are less likely to default and more able to meet their financial commitments.
2. The score leverages new data sources
As the market leader in consumer credit information, Equifax can tap into a wealth of data to build the quality and predictive ability of our scores. In Equifax Score, the latest comprehensive credit reporting data augments our extensive repositories of negative event and enquiry data. These new positive data elements provide increased signals of credit risk, further refining the accuracy of the score.
In the limited situations where there is insufficient data on an applicant’s credit file (thin files), such as new-to-bureau consumers, there is the option to supplement the score with alternative data. Geodemographic and Buy Now Pay Later are both available as additional data sets that add another layer to the score's existing indicators of creditworthiness. A best practice approach has been taken to incorporating these new data sets, including the use of de-identified data, restrictions on data collection and the adoption of privacy governance.
"When an individual applies for credit for the first time, the score doesn't use their age or gender, so not a lot is known about them beyond the financial institution they're applying to, the product they're applying for and their home address,” said Bruhn.
“Simply knowing where an individual lives allows us to feed geodemographic data into the scorecard. Based on categories showing the total proportion of credit enquiries in an area to the total proportion of defaults, we can get a proxy for the type of risk in the area this individual lives," he explains.
BNPL is another useful data set, with Equifax retaining the most contributors of BNPL data than any other credit bureau. With the emergence of new BNPL players and products over the last few years, increasing signals have built up in our data assets about what this profile looks like and how it relates to credit risk. We are now in a position to be able to leverage all this valuable data into our new generation score.
The new score can also use current enquiry data as an additional data source in situations where limited information is known about a borrower. So while current enquiry data has been removed from the score, an exception is made for new-to-bureau applications. In this instance, there is no discrepancy of score between lender and consumer because there is no score until the first time a credit enquiry is made.
3. Innovative techniques are used to extract more value from data
We now have new ground-breaking analytical techniques that reach wider and deeper into the data, pulling out more interactions than ever before.
Bruhn explains that where traditional logistic credit score models studied one variable at a time, machine learning allows us to look at multiple interactions with many variables. Because we don't know exactly how each variable will perform with all possible interactions, these interactions must be constrained, so the lender and consumer understand what's going on.
If a borrower exhibits behaviour such as paying bills on time and not applying for excessive unsecured credit, the model knows to push the score upward. Late payments and a lot of unsecured credit or applications for unsecured credit are interactions that drive the score downward. In this way we generate a monotonistic relationship that means the score will only ever increase or decrease with a certain behaviour. If we allowed the impact of a certain behaviour to both increase and decrease the score across the range of values for a particular variable this could lead to illogical outcomes that would not make sense to a consumer or lender.
This constraint requires the loss of some predictive power but the trade-off is vital to increasing the performance of the score and maintain a level of explainability, says Bruhn. "The explainablity of the score makes it deployable. There is little value in having a score that is the most predictive, but that cannot provide logical and actionable explanations for its outcomes.”
To achieve this balance, Equifax Score uses an explainable AI (xAI) machine learning scoring methodology known as NeuroDecision™ Technology (NDT). By applying this technology to our new generation credit score, we have been able to achieve both stability and power, while providing the distinct advantage of explainability.
This unique ability to better explain the elements of an individual score will foster trust in the validity of credit scoring, giving consumers the ability to understand how their credit behaviours influence the score outcome.
4. The score is designed to provide continuity during COVID-19 new normal
Building an optimal credit scoring model that enables a lender to accurately determine the risk level of an individual in challenging economic times requires a trade-off between predictiveness and stability. There is no value to a high level of predictiveness if the model is not robust and stable enough to weather future adversity. Equifax Score has been designed to provide lenders with a reliable tool of risk assessment that remains predictive in and out of economic downturns.
Developed to incorporate the learnings of COVID1-19, the new model relies less on recent default and comprehensive credit reporting (CCR) data that has been compromised by payment deferrals. In addition to recent data, the score taps deeply into a rich tapestry of data, including 24 months of CCR data and the full five years of negative enquiries and defaults.
"The model has been built to cope with borrowers who have recent information gaps in their credit file because it has less dependence on this information. It helps that Equifax has such extensive data assets to draw on as we have the largest collection of data, including CCR data, of any other Australian credit bureau," said Bruhn.
When the stability of Equifax Score was put to the test in a COVID-19 simulation, it came through with flying colours. The performance of Equifax Score compared to our previous generation score was analysed under the scenario where circa 30% of all consumers applying for credit had loans that were simulated as being in a payment deferral. In addition, all recent defaults over the prior 10mths were removed to simulate no defaults being loaded to their credit report. Both scores were robust, but Equifax Score showed its superiority with a Gini drop of only 0.9% compared to 1.6% for our previous generation score.
The results demonstrate the continuity of Equifax Score's performance and why it can be relied upon as an accurate assessment of risk – for borrowers of today and borrowers of tomorrow.
Migrate to our stronger, more predictive model to increase the precision of your lending decisions at every stage throughout the customer journey. Read more: The Next Big Thing in Credit Scores or email a One Score Sales Specialist to find out more.
1 When compared to using previous generation Equifax scores.
2 Under this scenario, we removed up to 30% of payment history data from all consumers applying for credit in the development sample and re-set accounts to ‘0’ to simulate a payment deferral or '.' missing if the loan was already in arrears to simulate a payment deferral that is now in hardship. All defaults were also removed leading upto the point of application. For both conditions this was applied 10mths prior to the date of score generation and performance measured against actual outcomes 12mths later.
Related Posts
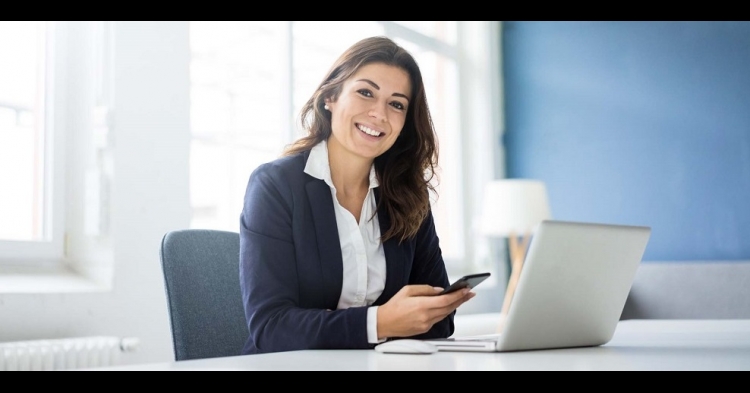
Businesses collect and process vast amounts of data, and often overlook the importance of ensuring the data is accurate, reliable, relevant, complete, consistent and timely. For a data-critical sector like insurance, the process of cleaning data to fix inaccuracies, incomplete, duplicate or wrongly formatted records holds valuable benefits.
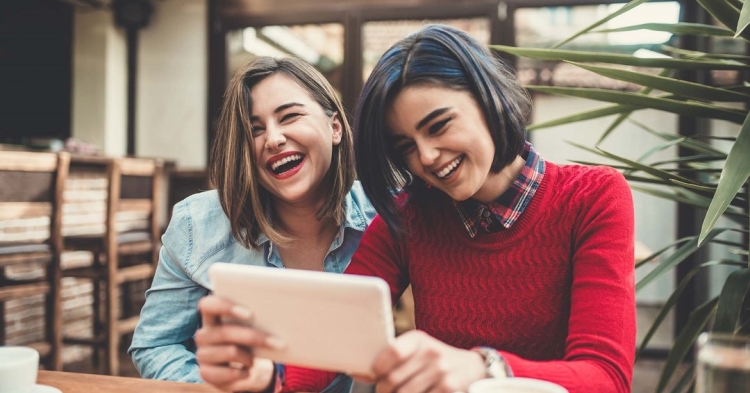
Establishing real, demonstrable trust in a person’s identity is essential for Australia’s 26.7 million people to transact online safely and effortlessly, levelling the playing field for credit access and fuelling our digital economy.